Normalized Wasserstein for Mixture Distributions
with Applications in Adversarial Learning and Domain Adaptation
Yogesh Balaji Rama Chellappa Soheil Feizi
University of Maryland
Paper | Code
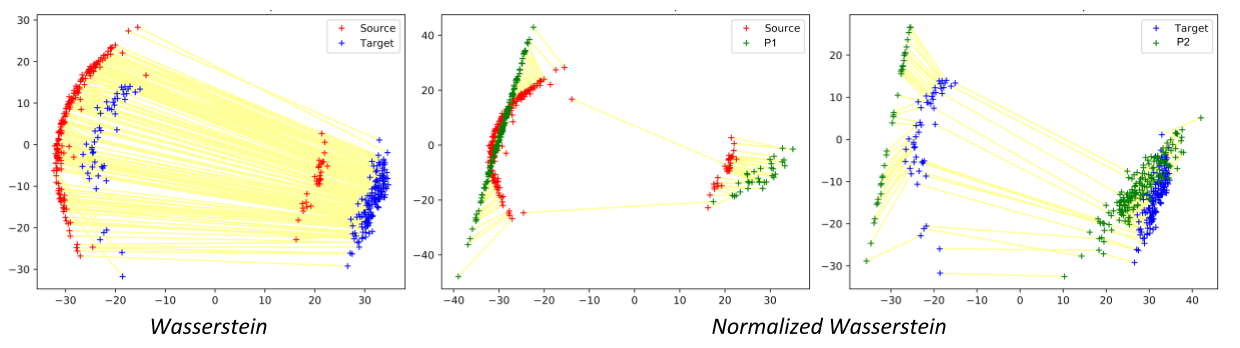
Abstract
Understanding proper distance measures between distributions is at the core of several learning tasks such as generative models, domain adaptation, clustering, etc. In this work, we focus on mixture distributions that arise naturally in several application domains where the data contains different sub-populations. For mixture distributions, established distance measures such as the Wasserstein distance do not take into account imbalanced mixture proportions. Thus, even if two mixture distributions have identical mixture components but different mixture proportions, the Wasserstein distance between them will be large. This often leads to undesired results in distance-based learning methods for mixture distributions. In this paper, we resolve this issue by introducing the Normalized Wasserstein measure. The key idea is to introduce mixture proportions as optimization variables, effectively normalizing mixture proportions in the Wasserstein formulation. Using the proposed normalized Wasserstein measure leads to significant performance gains for mixture distributions with imbalanced mixture proportions compared to the vanilla Wasserstein distance. We demonstrate the effectiveness of the proposed measure in GANs, domain adaptation and adversarial clustering in several benchmark datasets.
Paper
arxiv 1902.00415, 2019.
Citation
Yogesh Balaji, Rama Chellappa, and Soheil Feizi. "Normalized Wasserstein for Mixture Distributions with Applications in Adversarial Learning and Domain Adaptation", in IEEE International Conference on Computer Vision (ICCV), 2019. Bibtex